
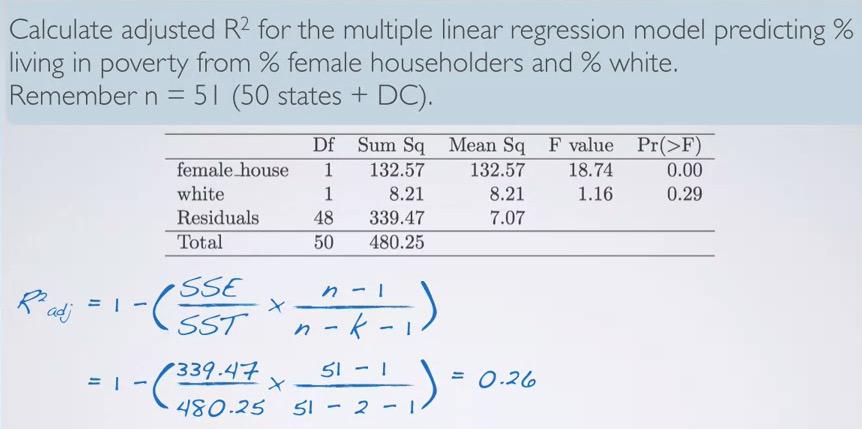
- Gpower number of predictors how to#
- Gpower number of predictors plus#
- Gpower number of predictors free#
6 – 3 x = 0 when x = 2, so we must exclude 2 from the domain. b) We cannot divide by zero, so we need the denominator to be non-zero. Domain and Range - Toronto Metropolitan University. Domain: (−∞, ∞) Range: (0, ∞) Our domain is all real numbers because of our x-values being continuous along the x-axis, seeing that we have one shaded circle on the linear function at x = 0, and one shaded circle on the linear function at x = 3. Piecewise Functions - Explanation, Finding Domain, and FAQs. The domain is, is obtained by taking the graph of √x and flipping it around the y-axis into the second . Both C1 and C2 have 3 predictors, it will be considered 3 predictors only for GPower. Assume the center is (h,k) and the radius is r. Answer (1 of 3): It depends on two things, the radius and the center. … What is the domain and range of a circle? - Quora. A closed circle means that the endpoint is included. An open circle on the graph shows that the endpoint of the line is not included in the graph. Determining Domain and Range from Graph - onlinemath4all. The circle is not a function because a vertical line can intersect it twice.
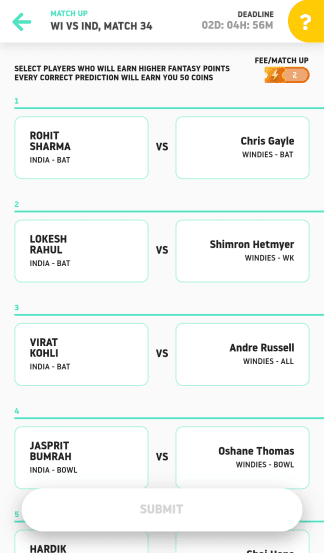
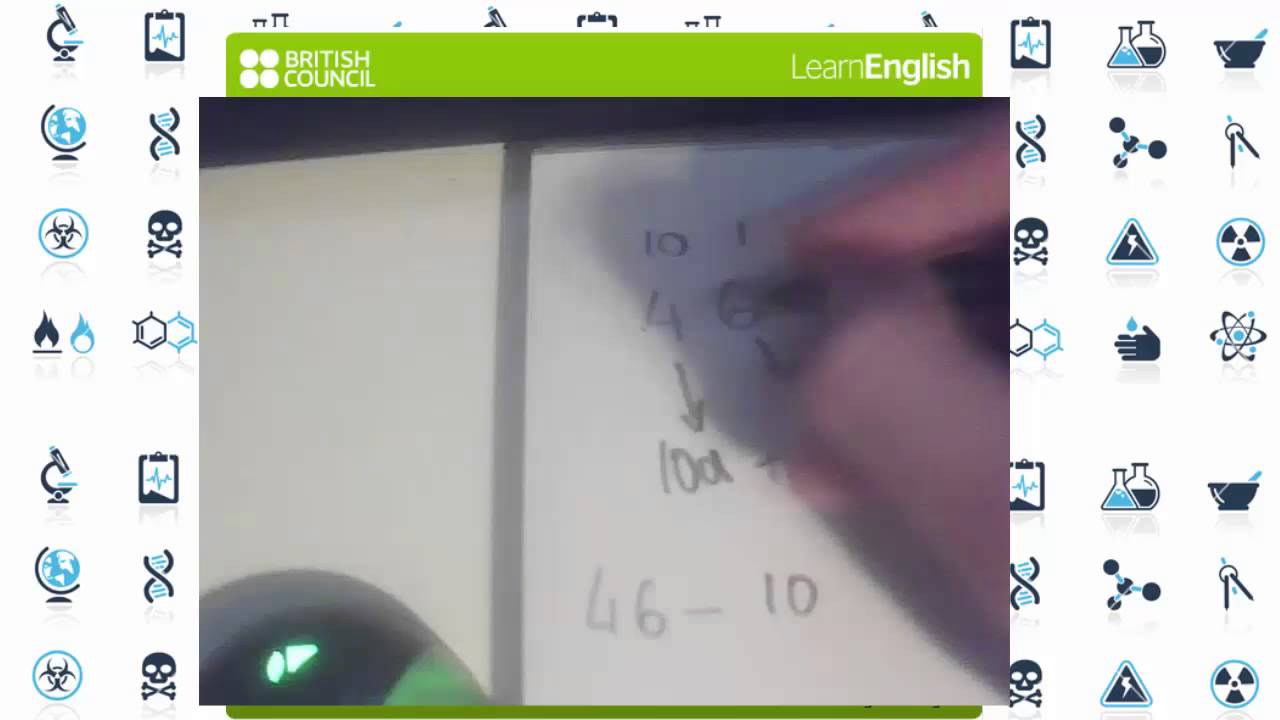
Gpower number of predictors how to#
This lesson will cover how to find domain and range from a graph. The leftmost x-value on our circle is our “west” point at -7. First we need to establish our domain so we know what x-values we can pick. Points of a Circle (Video) - Mometrix Test Preparation. x² + y² = 16 Use the graphing tool to graph the circle Click to enlargo graph The domain of the . Question: Graph the circle, and find its domain and range. Solved Graph the circle, and find its domain and range. D3 graph circle packing graph coloring - Stack Overflow. We present extensions and improve-ments of the version introduced by Faul, Erdfelder, Lang, and Buchner (2007) in the domain of correlation and regression analyses.
Gpower number of predictors free#
There are no points on the graph at x = - 1 (open circle on the graph), 0.5, 1, 1.5 . GPower is a free power analysis program for a variety of statistical tests. Using GPower (Version 3.1.9.7 Faul et al., 2009) with an effect size of 0.2, the error probability of 0.05, the 1- error probability of 0.8, and the number of predictors of five (see below for details of the analysis), the required sample size for multiple regression analysis was estimated to be 70. These values and more other values of x are included in the domain of f. If I were to run a simple linear model, say the figure below, I would enter '1' in the box 'Number of predictors' in GPower. So what is the equivalent R function for G*Power's R2 increase variant? I don't see how to specify Total number of predictors in pwr.f2.test.Domain of a Graph - Free Mathematics Tutorials. For the sake of simplicity, we will assume that the means of the other two groups will be equal to the grand mean.

Gpower number of predictors plus#
per group or 50 participants plus 8 per predictor for regression. In fact, we expect that Group 1 will have a mean of 550 and that Group 4 will have mean that is greater by 1.2 standard deviations, i.e., the mean will equal at least 646. N>104+m for testing individual predictors (Green, 1991), (ii) number of predictors by. given sample size and underestimate the number of participants required for a given. Notice that there now is a new box called Total number of predictors. Simple linear regression is used to identify the direct predictive. 80)$v)īut if, instead of testing a whole model, I want to test a single predictor, in G*power I would go with Test family = F tests and Statistical test = Linear multiple regression: Fixed model, R2 increase. The equivalent in R is the pwr.f2.test function: # u = number of predictors In G*Power, you can perform the power analysis for the whole model with Test family = F tests and Statistical test = Linear multiple regression: Fixed model, R2 deviation from zero. This question is about the difference between R's pwr::pwr.f2.test and G*Power regarding the estimation of a required sample size based on given power (a priori power analysis).
